- Orbital Insight Blog
- How to Choose The Right AI-Driven Geospatial Analytics Platform (Part 2)
Blog
How to Choose The Right AI-Driven Geospatial Analytics Platform (Part 2)
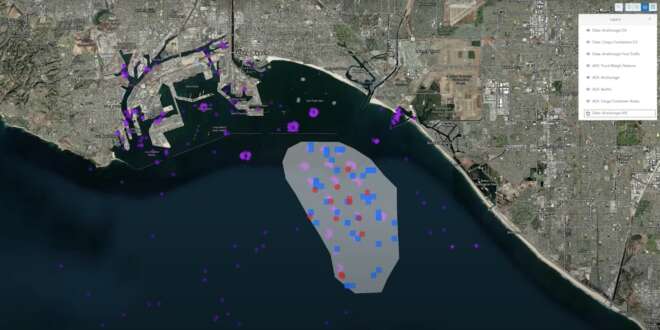
5 Tips for identifying capabilities, prioritizing features, and deciding which geospatial analytics platform is best for your business
Location data is transforming how businesses operate, particularly in Real Estate, Retail, and Financial Services industries. Geospatial data has quickly become a must-have resource to propel growth, drive revenue and address customer needs. So, how should you proceed if you have decided to augment GIS capabilities and enhance your organization's technology stack with AI-powered geospatial analytics? What are some of the technical features you need to consider? Which platform capabilities are most important? In the first part of this blog series, we presented an overview of the geospatial data workflow and outlined the common problems you will likely face when dealing with geospatial data. This second part gives you a rundown of the top five things to consider to help you narrow down and select a geospatial analytics platform.
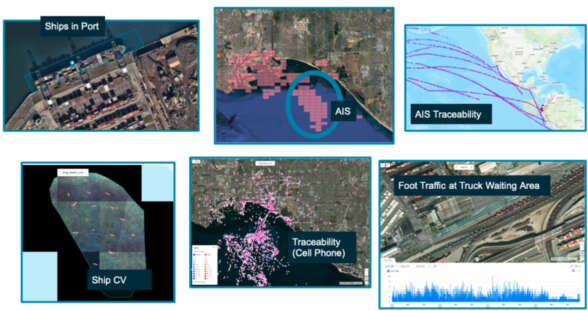
A platform capable of ingesting multiple data sources at scale will give the most accurate and in-depth analysis.
Evaluating A Geospatial Platform
An essential criterion for selecting the right geospatial intelligence platform is the end-users skillset. Do you have technical team members that are comfortable with data, GIS analysts, or data scientists in your group? How familiar is your team with coding basic or complex scripts and building visualizations? Here are the top five considerations to think through as you evaluate a geospatial analytics platform:
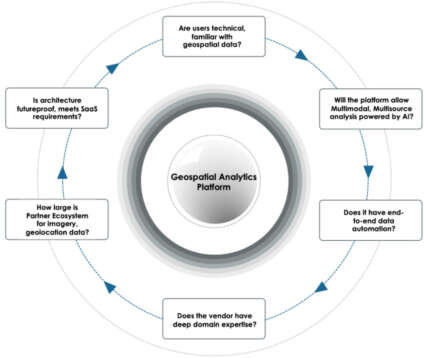
1. Multisensor, Multimodal AI
Geospatial data sources are growing exponentially - satellites, drones, ground-based sensors, maritime imagery, and other non-traditional data sources. To have the most accurate and in-depth analysis, you need a platform capable of ingesting multiple data sources at scale. The most valuable insights come from continuous real-time analysis and the fusion of data from various sensors to provide actionable insights. Several geospatial platforms are available today, and many platform vendors claim to offer a one-shop-stop experience. Unfortunately, most fail to deliver beyond basic analytics, can only do analytics on satellite imagery, and lack the capability for location analytics and vice versa. You need to look for a multi-modal, multi-sensor AI engine. For example, combining vessel data (AIS), geolocation, and connected car data can give you an accurate picture of what's happening at a port. RBC, the largest Canadian bank, is using geospatial analytics on 22 of the world’s largest and most influential ports. The analysis powered by the Orbital Insight GO platform suggests that the Ports of LA and Long Beach face historical challenges while the rest of the world is not. RBC’s Time of Turnaround metric, the time required for a container ship to enter the port boundary, unload, reload containers, and leave the port, has ballooned to 7.5 days for those two California ports versus pre-COVID norms of 3.5 days.
2. Automation to tackle data challenges
As any data science professional will tell you, raw data needs to be processed, cleaned up, and must go through transformation before it is ready for algorithms. Data quality is critical, so you need access to the best data sources available. Think hard about this step and avoid underestimating the data challenges. Will your platform be able to handle all the pre-processing and transformations required with imperfect geolocation data? Does it allow you to combine multiple geospatial data sets? Do you have an in-house data operations team to clean the data and perform corrections by removing noise?
3. Domain expertise in computer vision and location analytics
An AI-powered geospatial platform needs to combine active and archive imagery sources with computer vision algorithms, data science models, and cloud infrastructure. Building practical solutions to understand human activity requires strong domain expertise in various technical areas such as object detection, semantic segmentation, and statistics and leveraging several types of geospatial data to enable new use cases. This includes post-processing steps to compensate for potentially infrequent revisit rates in satellite data and normalization methods for addressing constant changes in sampling biases in geolocation data. As a geospatial analytics leader in your organization, you need to ensure that any platform you select will come with the necessary support. During the initial part of the project, the customer success team will need to provide additional guidance backed by valuable experience from past projects. In addition, you need to know about the accuracy of those algorithms. For example, can the vendors’ data scientists claim an F1 score of 0.98 for car detection algorithms and explain to you what that means? Finally, you need access to best-in-class AI capabilities. The best vendor should have experience with use cases relevant to your vertical such as detecting military movement, identifying foot traffic between multiple areas of interest, or picking the best location for your retail store.
4. Partner ecosystem to source sensor data
Working with geospatial analytics implies you need access to several different types of geospatial and other auxiliary data. That means you need to rely on a partner ecosystem consisting of multiple data providers - satellite imagery providers including EO, IR, and SAR, geolocation data providers, RF data providers, and providers of other data types such as demographics. The question to ask your vendor is, do they have an extensive collection of in-house datasets for image classification, object detection, land-use classification, and geolocation? Ideally, datasets need to come from different satellite sensor systems and geolocation providers. Datasets should also be adequately distributed geographically to address different use cases.
5. Future-proof architecture, SaaS infrastructure
Remember the platform you choose will have broad implications for the future. Your assessment needs thoughtful consideration of horizontal & vertical use cases, integration with other tools for visualization, and the ability to provide geospatial data analysis at scale. For example, will you be able to select one or multiple computer vision algorithms to detect, classify and characterize objects that you are interested in monitoring across large areas of interest? How about wide-area monitoring across states, borders, and countries? The right geospatial analytics platform will have an underlying architecture and core infrastructure that can support running computer vision and machine learning algorithms at scale, with historical data going back years and the ability to run in a continually-updated fashion.
Finally, integration and interoperability with existing infrastructure will be necessary. For example, the choice of enterprise data warehouse, machine learning capabilities, geospatial data formats, GIS platform, data lake, application latency, etc., are a few components that need to be considered as part of the assessment.
Do you want to monitor airfields, ports, and rail yards at scale? Need geospatial analysis on one to thousands of AOIs? Want to monitor trends and anomalies over days, weeks, months, or years? Have use cases that involve counting and analyzing cars, trucks, ships, foot traffic, tens of thousands of square km of land-use change in one platform? Then you need to check out the geospatial intelligence platform Orbital Insight GO: